Welcome to Part II of this series on payment models for healthcare AI! In this second installment, I define five usage-based pricing models, explore the strengths and weaknesses of each approach, and offer various examples of companies that have deployed these models in the wild.
If you haven’t read Part I, which explains why payment models are holding healthcare AI back, you can find it here.
The five usage-based pricing models discussed in this piece are:
Linear, Usage-based
Volumetric
Bundled Usage
Managed Services
Device-Maintenance
In Part III of this series, I’ll be diving into Performance-based pricing models for AI in healthcare. Subscribe below to receive it for free directly in your inbox.
Usage-Based
Linear, Usage-based
Definition: pay a flat rate per unit of value.
Linear, usage-based pricing is a business model popularized by API-first companies like Twilio, Auth0, and Sendgrid. The model has since found traction in healthcare with API-first companies such as Redox and Particle Health (clinical data interoperability), Lazarus (AI optical character recognition platform for document understanding), and Turquoise Health (price transparency data).
AI companies, especially those delivering value via inference through an API, can leverage canonical API business models to capture value for the underlying technology. For example, OpenAI leverages a linear, usage-based pricing model for the GPT-4 API based on prompt tokens. Determining the underlying unit of value (e.g., number of tokens, API calls, etc.) is one of the most important decisions AI companies must make when leveraging this pricing model. Determining how to relate value delivered to the pricing model is a multi-factorial problem that should always be rooted in an understanding of cost-to-serve (e.g., compute).
Example: Diagnostic algorithms, such as a computer vision algorithm that can detect diabetic retinopathy.
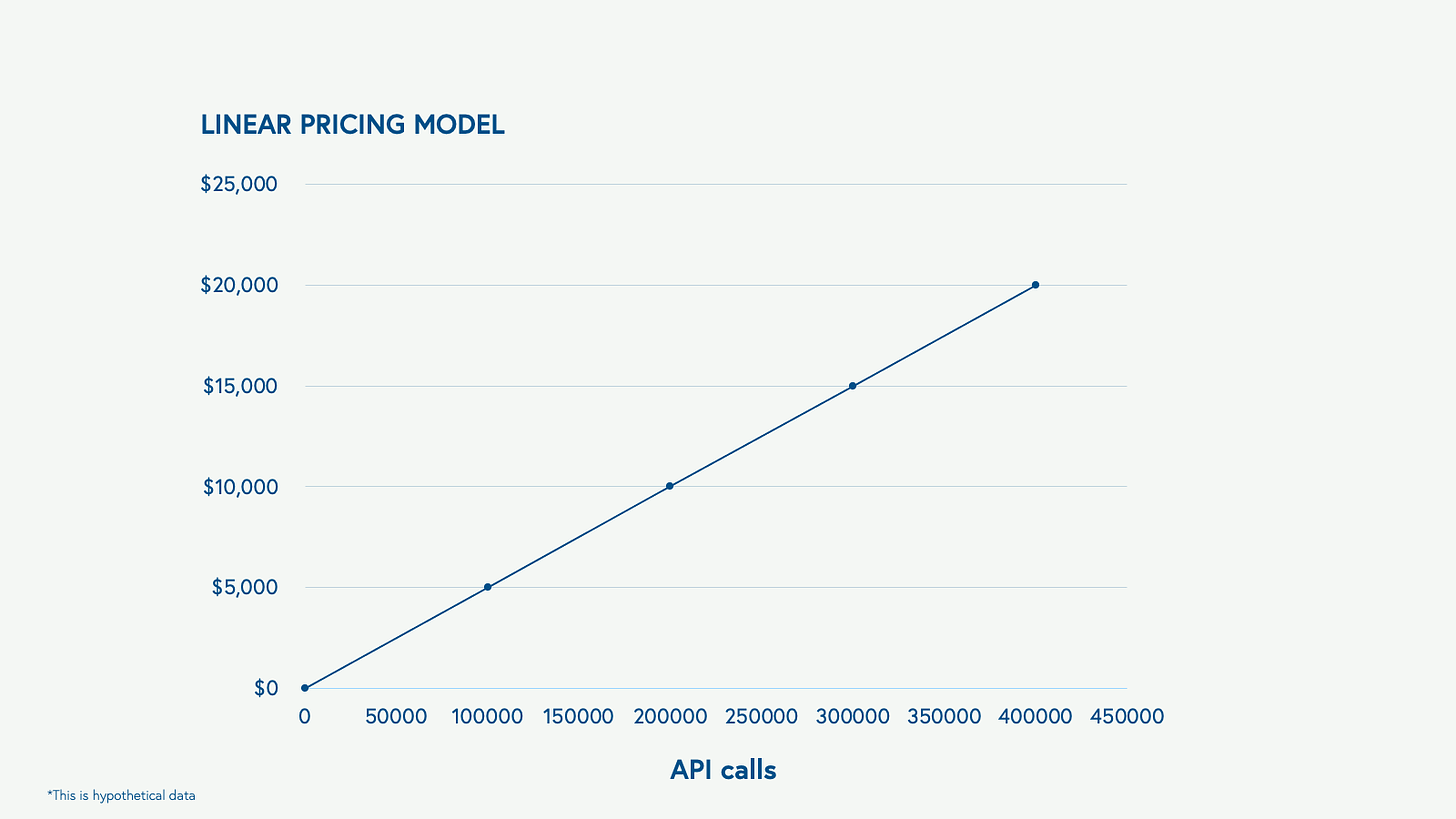
In healthcare, there are two primary ways to fund linear, usage-based pricing models:
1) Balance Sheet – customers pay directly for use of AI
As a conventional cloud business model, historically, balance sheet capital has funded linear, usage-based pricing models (i.e., the company benefiting from the AI pays for it directly from the vendor of AI); however, funding via this mechanism can pose TAM constraints within current healthcare and life sciences software budgets as described in Part I of this series.
2) CPT codes – health plan reimbursement for AI
The question of the day is: how do we tap into larger budgets for AI in healthcare, and specifically, payer reimbursement. Theoretically, health plan reimbursement for AI supported by linear, usage-based pricing models could dramatically expand the TAM, in addition to incentivizing utilization and access. Without proper billing infrastructure, there is little incentive (outside of “clinical goodwill”) to utilize diagnostic AI algorithms and manage the overhead associated with deployment.
I dream of a world where a new diagnostic algorithm drops onto a pre-print server or in a peer-reviewed journal, it is auto-evaluated by an independent governing body, and can immediately be downloaded and deployed by clinicians to serve patients.
Integrating these algorithms into the current reimbursement paradigms for diagnostics via CPT codes is one of the best approaches for aligning incentives for appropriate technologies. Of course, the journey does not stop with reimbursement, as validation, monitoring, clinical workflow integration, change management, and training are essential components, but are out of scope for this piece.
If it were as simple as assigning CPT codes to algorithms, barriers to deployment and adoption wouldn’t seem so steep. The truth is, CPT codes were never designed with AI in mind. Reimbursement amounts for CPT codes are driven by a complex set of inputs: relative value units (RVUs), geographic practice cost indices (GPCIs), procedure complexity and duration, specialty input, market demand, technology changes, among other parameters, all of which were instantiated in human-centric clinical services world.
As AI continues to flex compelling capabilities in diagnostics, it begs the question: How do we assign value to diagnostic AI under current reimbursement paradigms?
Working within the incumbent system, when we think about value capture for diagnostic AI, RVUs are an interesting starting point. RVUs are numerical values that reflect the relative resources required to perform the service, divided into:
Work RVU (wRVU): represents the physician's effort, time, and skill required for the service (a higher work RVU means more time and/or expertise from the physician).
Practice Expense RVU (peRVU): covers the operational costs of providing the service, including staff wages, equipment, and supplies.
Malpractice RVU (mRVU): accounts for the cost of malpractice insurance related to the service.
A brilliant study by Wu and colleagues found that, in the pricing for CPT code 92229 for diabetic retinopathy screening in the CMS fee schedule, “…despite having a relative value of 0 for physician work, the practice expense relative value (peRVU) for this code is 1.34, which is higher than that of its non-AI counterpart (CPT code 92228, peRVU=0.53). This difference illustrates how the pricing of AI devices shifts some of the value typically assigned to physicians toward the costs of purchasing and operating the device itself.”
The study also identified notable inconsistencies in reimbursement for AI compared to non-AI powered diagnostics across government and private payers, without an apparent “Goldilocks” model for rate determination:
Discrepancies between public and private payers: In the case of CPT code 92229 for diabetic retinopathy, the privately negotiated rate was 2.8x that of CMS ($127.81 vs. $45.36).
Comparable reimbursement for AI vs. non-AI diagnostics: Reimbursement for AI interpretation of breast ultrasound (CPT codes 0689T–0690T) had “a median negotiated reimbursement rate of $371.55, which is comparable to the national average cost of a traditional (non-AI) breast ultrasound of $360.74.”
Materially higher reimbursement for AI diagnostics: Analysis found that median negotiated rates for AI-powered cardiac CT for atherosclerosis was $692.91, which is significantly higher than the average cost range of $100.00 to $400.75 for a cardiac CT without AI.
Overall, the data is inconclusive as to whether AI diagnostics should be more or less expensive than non-AI counterparts. In many instances, such as image-based modalities, AI isn’t necessarily making an exam cheaper, but is instead:
1) offering additional insight imperceivable by the human interpretation, or
2) improving quality by reducing inter-reader variability
If the goal is to take an RVU-based approach, more research is required to calculate all three components across physician work, practice, and malpractice expenses.
The success of linear, usage-based pricing models for AI in diagnostic settings will require us to determine the value of exams with AI compared to non-AI based technologies. “Value assignment” remains an area of further work and investigation.
3) Consumer Cash Pay – charge out-of-pocket fees to consumers for AI-powered services
Another option is to pass fees for AI onto patient-consumers as an add-on service. RadNet, a large diagnostic imaging services company, launched a program that charges patients a $60 out-of-pocket fee for an AI mammography read. About eight months into the program, RadNet reported that 20% of patients opted to use the add-on AI service.
Below is a non-exhaustive list of the advantages and disadvantages of linear, usage-based pricing models:
Volumetric
Definition: purchase an allowance based on expected product use, potentially pay overage fees if actual usage exceeds the allowance.
In a volumetric model, customers buy an allocation according to their anticipated product usage. Subsequently, when the customer surpasses this usage allowance, the pricing structure undergoes a modification. Typically, this adjustment manifests as either a discount or an additional fee for exceeding the allotted usage. Some businesses adopt a similar approach where the cost per unit decreases as the volume of usage increases. Many API-first companies have pioneered volumetric models as improvements to usage-based as it incentivizes greater utilization.
Example: Hospital buys bulk GPT-4 API access for powering asynchronous message drafting for clinicians under a fee structure that decreases as message volume increases.
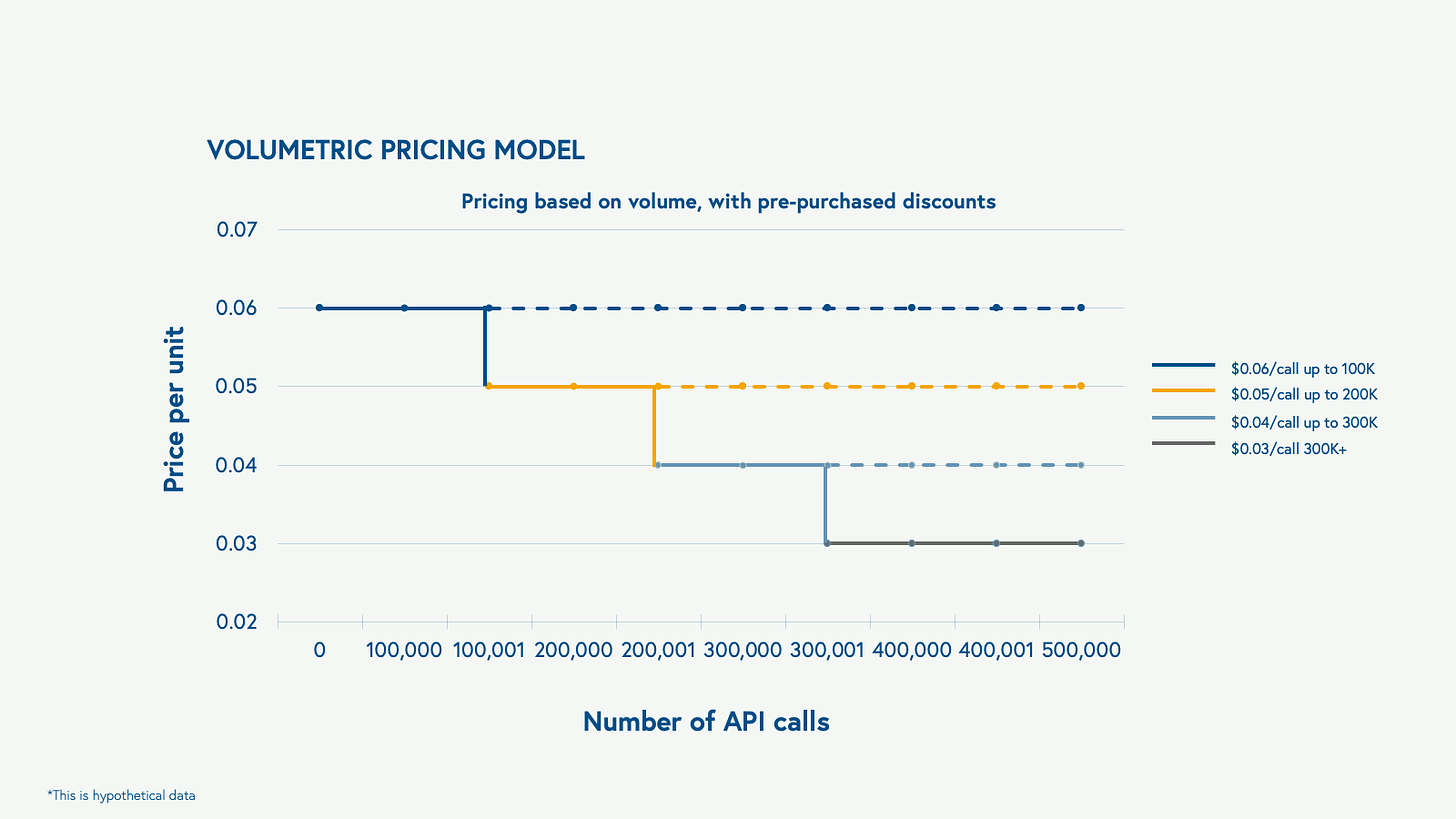
Below is a non-exhaustive list of the advantages and disadvantages of volumetric pricing models:
Bundled Usage
Definition: a tiered usage-based model with additional features at higher prices.
In a bundled usage model, the price per unit of usage escalates in higher tiers to encompass additional features.
Example: AI scribe charges per clinical encounter, and charges more per encounter for premium features such as reduced inference time (e.g., real-time) or additional capabilities such as discharge summary generation or medical coding.
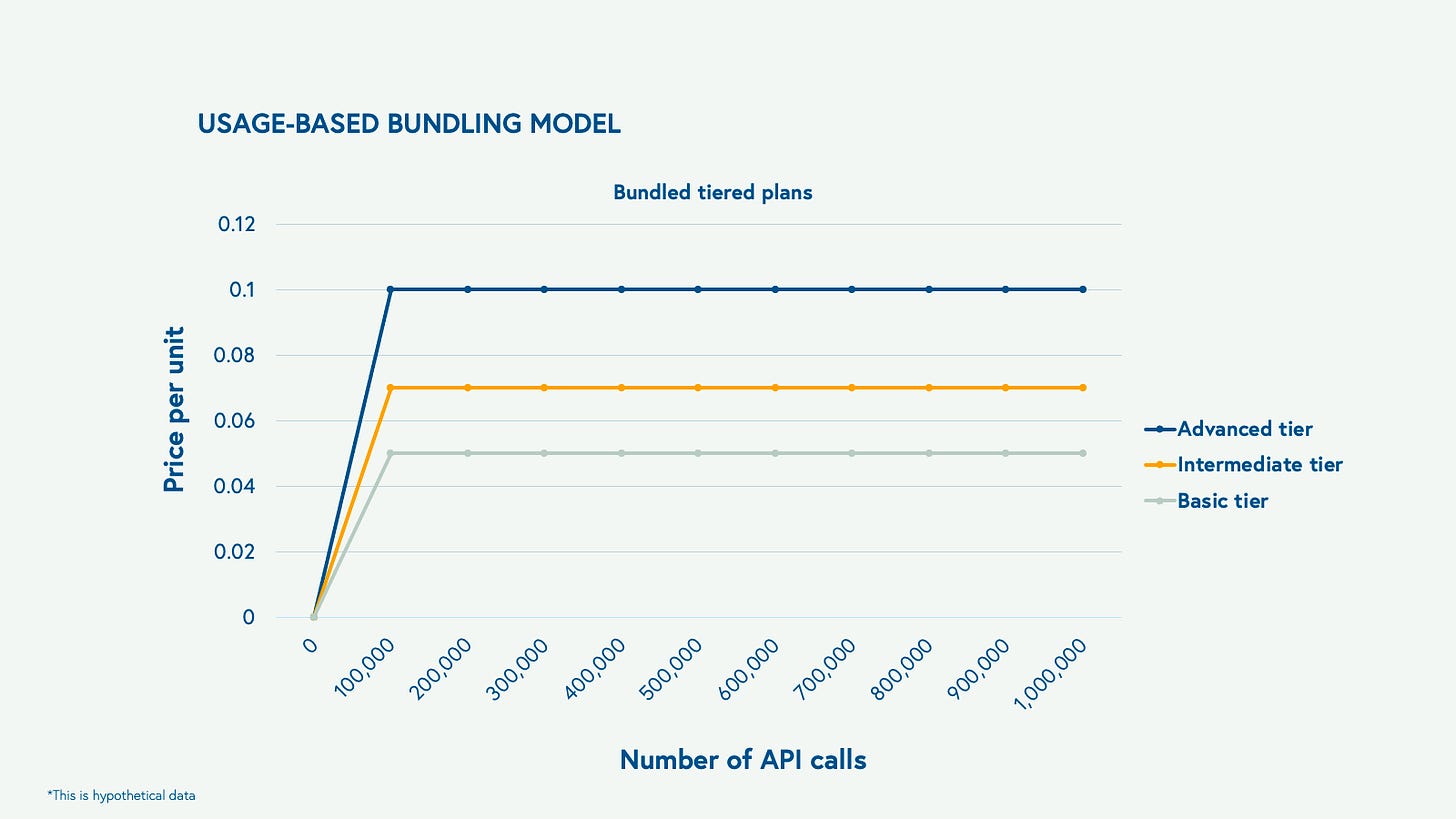
Below is a non-exhaustive list of the advantages and disadvantages of bundled usage pricing models:
Managed Services
Definition: pay recurring fees for a continuous outsourced service.
A managed services business model provides proactive, outsourced management of specific functions through subscription-based services. Customers benefit from predictable costs, scalability, 24/7 support, and expertise, while mitigating risks associated with managing their own systems. There is a long history of managed services business models in healthcare in the form of physician practice Managed Services Organizations (MSOs), outsourced hospital IT, and revenue cycle management. Most of these incumbent businesses are powered by people who are deployed onsite equipped with domain expertise, technology, or both.
While these individuals may work at a clinic, health system, payer, or biopharma company, they remain employees of the parent organization. I think of managed services programs as a subset of business process outsourcing, though the distinction between the two is not essential for this piece.
With autonomous AI agents, there is an opportunity to build large, highly scalable managed services offerings that can scale non-linearly with limited-to-no human resources. My favorite definition of an autonomous agent from Matt Schlicht’s blog:
“Autonomous agents are programs, powered by AI, that when given an objective are able to create tasks for themselves, complete tasks, create new tasks, reprioritize their task list, complete the new top task, and loop until their objective is reached.”
Autonomous agents represent a step function in abstraction – meaning, the removal of humans in directing small sets of tasks frees up resources to manage at higher levels and in a more scalable fashion (until, of course, models are simply managing other models). The result is that the output of a system is no longer that generated by the user leveraging said system, but is the work product generated by the system itself.
More simply, as Sarah Tavel wrote on her blog, “AI startups sell work, not software.”
The phenomenon of selling work calls for a shift from building technology that supercharges an existing workforce to technology that delivers the work product itself.
Examples:
AI autodialers that are offering “Contact Center-as-a-Service.”
Electric AI, an automated outsourced IT vendor, is an example of a next-gen AI-enabled company that leverages a managed services business model by charging per employee per month recurring flat fees.
Stripe is launching AI-powered Support-as-a-Service.
In truth, managed services businesses can have a diverse array of underlying fee schedules that inform monthly costs, and of course, the monthly fees can be variable. Managed services can invoke linear, usage-based business models based on the number of work units delivered, as well as volumetric or bundled versions. Value-added services like training, consulting, or data analytics may be offered separately or bundled into the monthly fees.
Re-architecting the cost structure of managed services is especially exciting in healthcare and life sciences – industries run by swarms of outsourced entities. In recent news, health systems are increasingly outsourcing their IT departments to managed service companies to enhance efficiency, save costs, and scale up initiatives such as virtual care, artificial intelligence, and analytics. This strategy, known as rebadging, involves transferring analytics and tech employees to external managed services firms.
The article provides two examples:
Care New England transitioned its IT department to Kyndryl to enhance efficiency and focus on core healthcare services, leveraging Kyndryl's expertise in managing IT operations.
Carle Health collaborated with Health Catalyst, aiming to improve its data analytics and patient care capabilities.
“More hospitals are going to “workforce on demand” models, where you treat your IT workforce like you do the cloud. It scales up and down and you can bring in resources when you need them.” -Erik Pupo, Guidehouse’s Commercial Health IT Advisory Director
While the managed service approach offers financial benefits and allows clients to focus on “bread and butter” workflows (e.g., clinical work for hospitals), it can also present challenges around potential quality issues and negative impacts on employee morale. As we deploy more autonomous agents for managed services, we’ll learn more about these boundaries.
What we do know: Well-designed autonomous AI agents and “workforces-on-demand” will compete at a fraction of the cost, work 24x7, and produce results that are just as good if not better. These systems will move us beyond the tired “co-pilot” model to “full auto-pilot,” tackling AI’s Faster Horse Problem head-on. In healthcare, it’s essential that we determine where these approaches are best deployed (i.e., non-clinical vs. clinical) and organize accordingly.
Below is a non-exhaustive list of the advantages and disadvantages of managed services pricing models:
Device-Maintenance
Definition: pay an upfront cost for the AI product (or recurring leasing cost) plus annual maintenance fees.
The Device-Maintenance pricing model solicits an initial upfront fee plus a recurring leasing fee for acquiring the AI product, accompanied by annual maintenance fees ensuring continuous functionality, performance, and support. These fees cover regular updates, patches, bug fixes, and technical assistance, while optional upgrades and enhancements may be available for an extra fee.
Under this model, service-level agreements define the support standards, and customization or integration services may incur additional charges. Scalability is accommodated to adjust usage levels and costs, promoting transparency and predictability in billing. Like the Managed Services model, value-added services such as training, consulting, or data analytics may be offered separately or bundled into the maintenance fees.
Example: Hardware-based medical devices often offer devices on a purchase, lease, or rental basis, charging maintenance and upgrade services over time.
Below is a non-exhaustive list of the advantages and disadvantages of Device-Maintenance pricing models:
Hope you enjoyed my pontification on usage-based pricing models for healthcare AI.
In Part III of this series, I’ll explore five performance-based models in detail:
Subscribe to receive Part III for free directly to your inbox:
Thanks for reading!
Morgan
Huge thanks to my pals Nikhil Krishnan and Gaurav Singal for their feedback on this piece.
I also drew inspiration from this excellent post by my colleagues at Bessemer.