Healthcare AI Thesis Update
This is not a market map – 4,000 words exploring the research, products, business models, and market entry points that are reimagining healthcare with AI
This piece was originally published on Bessemer’s Atlas, co-authored by Steve Kraus and the Atlas Editors.
Healthcare AI is at a tipping point, with research breakthroughs and innovative business models unlocking unprecedented opportunities that are reshaping both markets and modern medicine.
AI in healthcare is an overnight success 80 years in the making. As the underlying technology matures for real-world applications, companies reimagining this industry are being met with unprecedented demand.
Healthcare AI is having its moment, with an explosion of new entrants and exceptionally high market demand for solutions from established incumbents. We are seeing this play out in the startup ecosystem and across boardrooms at Fortune 500 companies, as CEOs from within the industry and outside are eyeing healthcare AI as an immense market opportunity — from Big Tech and Big Pharma, to the very institutions that are responsible for our own care.
While the hype might seem like a repeat of past waves of short-lived techno-optimism in healthcare, the data tells a different story. In one recent survey, 70 percent of payers and providers reported pursuing implementation of generative AI. Three-quarters of these same groups have increased IT investments over the last year and expect this trend to continue. And nearly two-thirds of biopharma executives are actively building out use cases across commercial and research and development (R&D) applications, while 60 percent have set near-term targets for expected return on investment.
The foundation for the healthcare AI revolution has been laid by previous technological breakthroughs in the industry. Decades of investment in driving electronic health record (EHR) adoption and digitizing clinical and administrative data have turned healthcare into a fertile ground for the development of AI applications, with troves of text, images, videos, lab values, and other types of data now machine-readable and available as fuel for AI systems.
Several conditions have made this unique moment possible:
Underlying technologies have finally matured for real-world applications, underscored by the fact that the nearly 40 percent of healthcare technology startups that raised capital in 2024 are commercializing AI technologies.
Stakeholder sentiment is shifting dramatically, with nearly three-quarters of healthcare leaders and two-thirds of physicians expecting AI to significantly improve aspects of workflows spanning administrative to clinical use cases.
The healthcare industry needs innovative regulatory and market solutions to restore its economic sustainability. Hospitals are grappling with inflationary costs and financial instability, payers are navigating rising medical loss ratios, and biopharmaceutical companies are facing diminishing R&D returns. Considering these challenges in light of the rapid advancements in AI, it’s obvious that mature, adaptive regulation is not an option — it’s an imperative.
Re-platforming healthcare will not be driven by a single solution, but by a confluence of inventions, with AI emerging as a particularly potent catalyst. This moment has been built on decades of pioneering work in the artificial intelligence and informatics fields. Starting in the 1960s and 1970’s, the healthcare field inspired pioneering work in informatics and methods research, a precursor to the eras of predictive AI, deep learning, and generative AI that followed in the 2000s (as shown below).
There’s now a clearly defined path for translating AI research into sophisticated products and services that are ready for use in healthcare settings — not too dissimilar from the mechanisms that facilitate research-driven innovation in the biotech industry. There’s even entirely new academic journals, such as NEJM AI, that have emerged to bridge the gap between AI research and implementation. Turning high performing models into commercial products won't automatically result in real-world change. Figuring out the right delivery method and business model for AI models, and positioning them in markets where there are high unmet needs will help translate AI healthcare research to real industry and patient outcomes.
Since funding is a lagging indicator for emerging technology, the landscape we’re seeing in 2024 is likely just the tip of the iceberg. As AI research in healthcare continues to accelerate, we predict that startups and incumbents will continue to benefit over the next three to five years. Moreover, as the market gains a deeper understanding of AI's potential and limitations in healthcare settings, we expect to see increasingly refined, novel, and high-impact solutions coming to market.
Six years after the initial launch of our Deep Health investing thesis, we share our updated thinking in healthcare AI. In this roadmap, we’ll discuss three of the primary drivers of new opportunities in healthcare AI and formidable challenges AI companies may face when operating in this vertical. Building upon our six imperatives for AI-first companies, we’ll also offer strategies for overcoming these hurdles and carving out a path to defensibility, with a focus on healthcare AI modalities, business models, and market opportunities.
Three drivers of opportunity for healthcare AI
1. Healthcare produces 30% of the world’s data — and now it’s digitized
Healthcare generates an estimated 30% of the world’s data, growing faster than other data-rich industries such as finance and media. This statistic has been shared widely, but what often gets left out is that up to 97% of data generated by hospitals goes unused — an inefficiency that points to an immense, largely untapped wellspring of data that could be used to help improve or even reshape healthcare operations and patient care.
In the 2010s, following a multi-decades-long process and a strong regulatory push, much of healthcare’s clinical data finally became digitized through EHR adoption. As a result, a treasure trove of data in the form of clinical records (including text, images, lab values) became machine-readable for AI. Paradoxically, and despite the industry’s near-term focus on automating back office tasks and workflows with using AI, much of the administrative data in healthcare remained on paper longer, largely because the regulatory catalysts to digitize this information came later.
The eventual digitization of healthcare data across functions also accelerated cloud adoption in healthcare — a critical foundation for the successful implementation of AI applications. Compounded by the dramatic increases in model sizes and compute as well as the development of new multimodal architecture, healthcare’s ongoing digital transformation has made this vertical fertile ground for AI companies. Companies like SmarterDx are capitalizing on this trend, empowering hospitals to close revenue integrity gaps and improve quality metrics leveraging their own data. Plenful, a pharmacy-focused workflow automation platform is unlocking value by applying AI to customer data across 340B auditing, document processing and inventory planning, and prior authorization tasks.
2. Healthcare AI is re-defining our understanding of health from the proteome to populations
After years of progress, it’s now possible to model a patient’s health from the function of a protein all the way up to the populations to which that patient belongs and the systems they interact with to access medical care. Each day, more data and novel types of data become available, as providers adopt new molecular assays such as single cell sequencing and medical devices capable of measuring new health signals. Regulation has also continued to bring new data types online. Turquoise Health is a platform bringing hospital price transparency data to market on the back of the recent CMS Hospital Price Transparency Rule.
Wielded effectively, all of this data can paint a multi-dimensional view of healthcare, and be used to develop AI products and services addressing a wider range of complex biomedical and healthcare use cases. The newfound ability to combine and run models on multiple datasets has opened the door to even more possibilities, the number and scope of which have not yet been fully understood. This is one of the biggest reasons we consider healthcare a “holy grail” for the development and application of multimodal AI.
3. Healthcare AI research is translating into products and services
Research in healthcare AI began increasing in the early 2000s, beginning with predictive AI, then deep learning, and eventually generative AI. While much of that research uncovered insights and led to the development of models that could have benefited healthcare, it didn’t reach its full potential at the time because healthcare organizations didn’t have a robust way to put those models into practice at scale. But that’s all changing. Decades of research are now being translated into tangible AI products and services that are “ready for primetime.”
Just look at the exponential growth in FDA-approved AI/ML-enabled devices since 2000, with 30x as many approved devices in 2024 than in 2014.
We’ve now reached a critical inflection point in healthcare AI. Today, each new breakthrough in research has the potential to solve real-world problems — an opportunity that should galvanize founders and investors to engage more deeply with emerging developments in the field.
Challenges for healthcare AI
There are still countless obstacles for AI startups in healthcare despite the promising new developments, early success stories, and a growing set of possible use cases. Making an enduring impact will require exceptional resourcefulness and ingenuity from founders and their teams. Here, we outline some of the greatest challenges for the emerging class of healthcare AI companies, and offer strategies for mitigating the effects.
1. Traditional market forces don’t apply in healthcare, but physics does
The US healthcare system is notoriously hard to navigate. Bound by numerous and ever-changing regulations and a complex interplay of often misaligned incentives, this sector operates in defiance of conventional economic principles — leading to a tangled web of dependencies that looks something like this:
While the US healthcare system may defy classical market economics, it adheres rigorously to Newton's Third Law: for every action, there is an equal and opposite reaction. Healthcare AI founders need to be aware that their companies are not immune to these dynamics, and that, in the era of AI, resistance to change will likely emerge more quickly and with greater intensity. Look no farther than what some are calling the impending “payer-provider” algorithm war. If you build an AI-powered diagnostic tool that is billed for reimbursement with every use, for example, you should expect health plans on the other side to deploy AI to interrogate what is appropriate use. In this sense, the traditional checks and balances of healthcare are also being supercharged by AI, creating a faster-paced environment where innovation and pushback evolve in lockstep.
2. Regulation is both a barrier to entry and an enduring moat
Healthcare is a tightly regulated industry where the law tends to protect the status quo. The barrier to entry for startups to get to market can be sky high, and for companies operating in areas of healthcare where regulations are indeterminate or gray such as AI, this is even more true. The dense regulatory landscape that creates obstacles for newcomers doubles as an enduring moat for the old guard — a small group of deeply entrenched healthcare incumbents that wield immense power and influence over the industry.
It’s unsurprising that the position of these incumbents in healthcare is ossified. A recent internal analysis revealed that top U.S.-headquartered healthcare companies by market share have a median founding year in the 1960s. The US healthcare system is obviously very different from it was then (with new regulations, adoption of technology, exploding costs of care, development of curative therapies, etc.), and yet incumbents have been able to ride the waves of change and maintain dominance — and by a large margin. Several healthcare incumbents have retained a market share of 70%+. For comparison, on-demand cloud computing’s category creator and leader, AWS, commands just under a third of the market.
Healthcare AI has become a focal point for US policymakers and legislators, prompting a fundamental reassessment of long-standing healthcare regulations and principles, including cornerstone policies like HIPAA. Dynamic regulatory environments can bolster incumbent footholds and compound the challenges for startups, so founders and teams should not only track the changing landscape and be ready to adapt, but consider actively engaging via advocacy and lobbying work.
In some unique cases, regulation can be a catalyst for new company creation and proliferation, for example: Datavant, with HIPAA and health data interoperability rules, Collective Medical, with the HITECH Act, and Veeva Systems, with the Physician Payments Sunshine Act.
But whether a law is providing opportunities or restricting them, deeply understanding the regulatory landscape is a must, and even when not constrained by regulation, healthcare AI companies should always center in safety and ethics in model development and business practices.
3. Markets are smaller than they appear
Healthcare is one of the largest industries in the US with expenditures accounting for 17% of the GDP and employing 1 in 10 American workers. It’s also wildly inefficient, with $1 trillion spent annually on administration-related costs. To put this into perspective, the time practitioners spend billing insurance can account for $1 in $7 of revenue for the services billed, in some cases.
While healthcare is technically a $4.5 trillion market, the industry is far from a monolith. We’ve come to view the healthcare market as a container for ~4,500 distinct markets, each with a TAM closer $1 billion; or, ~1,000 unique markets, each with a TAM of $4.5 billion. For simplicity, we’ve grouped the high-level market segments below:
As you can see, clinical services (hospital care, physician services, clinical services) represent more than half of the overall healthcare market, but few healthcare AI companies have tapped into this spend directly (companies like Hinge Health, Headspace, and Ansible Health are making strides). On the other hand, while drug discovery remains a highly active area for AI companies, the TAM of any one AI-first biotech company is a far cry from the $406 billion prescription drug segment, as biotech market sizes depend on the patient population and availability of existing treatments for a given disease.
So who exactly will pay for AI in healthcare? After all, half of US hospitals were margin negative in the wake of the COVID-19 pandemic, and the average hospital spends just $7.8M a year on IT (including electronic healthcare records, security, and personnel). This is also an industry where budgets are heavily allocated towards services (especially, labor), and software that improves workflows or increases productivity is only worth so much.
For success in any market, unlocking sufficient TAM is essential and it can be especially tricky in healthcare. Still, we’ve already witnessed a new generation of healthcare AI companies that are forging ahead. We’re seeing teams adopt innovative modalities and business models to tap into bigger budgets and multiple buyers, allowing them not just to survive but to thrive, and even access TAMs that were unthinkable in the previous wave of what we used to call “healthcare IT.”
Our theses on healthcare AI
Our analysis of AI's ongoing impact on healthcare has led us to formulate three key hypotheses that inform our approach when evaluating potential investments in this rapidly evolving sector.
1. Modality-business model-market fit determines value creation
The modality of an AI solution and the company’s business model can have a significant impact on available TAM and gross margins. By modality, we are referring to the way an AI product is delivered (e.g., software, copilots, agents, services, diagnostics, therapeutics). Companies addressing the same or similar workflows or sub-verticals in healthcare via different business models can have a TAM differential of as much as 25x (as we’ll show in the example below). It’s no exaggeration to say that modality-business model-market fit is a make or break for venture-backed healthcare AI startups. Let's consider the options:
Modalities
Vertical AI modalities
AI-enabled Software: A platform enriched with AI capabilities to improve functionality and user experience
Copilots: AI-enabled assistants that work alongside a user to enhance productivity by automating tasks within an existing workflow
Agents: Autonomous or semi-autonomous systems that perform specific tasks with minimal human intervention
AI-enabled services: Traditional services augmented with AI capabilities to improve efficiency, accuracy, or user experience
Healthcare AI-specific modalities
Diagnostics and therapeutics are modalities unique to healthcare. AI diagnostics support or automate the identification of disease through existing or new paradigms (e.g., AI for retinal imaging has been applied for diagnostics across cardiovascular, metabolic, and neurologic diseases) and can be reimbursable by cash pay, insurance, or enterprise contracts, such as in the context of AI biomarkers for clinical trials. Going to market with a clinical diagnostics tool is often more arduous and time-consuming than with other modalities because diagnostics require both regulatory approval and the trust and evangelism of practitioners, who (rightly) may be skeptical of AI operating within such a high-stakes area of patient care. But it’s not impossible, and there are creative ways to get to clinic. Some AI diagnostics companies have already overcome these barriers, including Cleerly in cardiology, Viz.ai in neurology and cardiovascular, Digital Diagnostics in ophthalmology, and Tempus in oncology.
The other healthcare-specific modality is therapeutics, medical treatments designed to alleviate symptoms or cure disease. While there’s potential for companies to build AI-powered digital therapeutics that treat certain conditions — as we have seen with video game-style therapeutics for improving attention in children with ADHD — there are serious challenges related to adoption and reimbursement for this type of use case, and requiring stronger incentives to drive widespread uptake. The more common use case for AI in therapeutics is to aid in drug discovery and development. For example, Bessemer-backed Seismic Therapeutic is using machine learning and AI in drug discovery and development for autoimmune diseases, tackling key challenges such as mitigating immunogenicity.
In reality, companies will combine multiple modalities within a comprehensive solution. For instance, Qventus provides perioperative optimization solutions via software and agent-based services.
Business models
The modality of an AI product can help inform the best business model. While multiple business models can be deployed in tandem, we’ll define each one individually for the sake of simplicity. At a high level, there are two primary business model architectures: usage-based and performance-based.
For a deeper dive on some of these business models, read our Atlas piece on SaaS pricing models, as well as these deep dives on usage-based and performance-based models in healthcare.
Effects on TAM and gross margin
To illustrate the effects of modality and business model on a company’s TAM and gross margins, we’re using an example of a hypothetical startup innovating in the ophthalmology space.
For context, there are roughly 20,000 ophthalmologists and 50,000 optometrists in the US, 18 million retinal images taken every year, and seven million ocular injections made per year. In the 2010s, researchers developed a method of using retinal images to identify pathologies in areas other than the eye such as the precursors to diabetes. Our hypothetical startup has created an AI solution catalyzed by these developments.
Depending on the modality and business model, the TAMs listed above range from $84 million (for a copilot product with a pay-per-seat SaaS model) to $2.1 billion (for an AI-enabled service that aids in ocular injections with a usage-based or “fee for service” pricing model).
As this example highlights, AI models, when deployed in isolation, often fail to capture their full potential value and can present adoption challenges for customers. To maximize value capture and facilitate smoother adoption, companies should consider vertically-integrated business models that use AI to enhance or reimagine existing workflows, effectively meeting customers where they are. These vertically integrated approaches not only solve more comprehensive problems but also tap into larger revenue streams across the value chain, thus expanding the addressable market size.
Consider the following examples of a targeted software solution and its vertically-integrated counterpart:
Radiology: AI-first radiology software product vs. AI-enabled radiology services provider optimizing the entire image acquisition and interpretation workflow
Revenue cycle management: AI-first coding and billing software vs. comprehensive revenue cycle management service with AI features and capabilities
Clinical trials: AI-first software for clinical trial recruiting and site selection vs. full-service agency that manages end-to-end trial recruiting and site selection using AI
Drug discovery: AI-as-a-service provider for drug discovery companies vs. integrated drug discovery company leveraging AI to develop therapeutic programs
It’s worth noting that modality and business model can affect gross margins too, and there are often trade-offs between higher TAMs and healthier gross margins. For example, while the AI-enabled ocular injections service has a TAM of nearly twice that of the AI software product, SaaS has historically supported much higher gross margins than services in healthcare. But this convention is being challenged by AI companies, many of which are using AI to increase the efficiency of services-based delivery models.
2. Multimodal AI is the holy grail for healthcare
Advances in AI have produced powerful models that excel at a variety of tasks across data types, such as text and images. Until recently, most of these models had been built for singular data types. Given that healthcare data is multi-dimensional — encompassing clinical records, medical imaging, audio, video, patient-reported outcomes, wearable device data, time-series information, exposure data, genetic sequencing results, and more — healthcare AI is fertile ground for the development of and application of multimodal architectures, inspiring the creation of entirely new fields that work at the intersections of a wide-range of data modalities and applications, from radiogenomics to operational informatics.
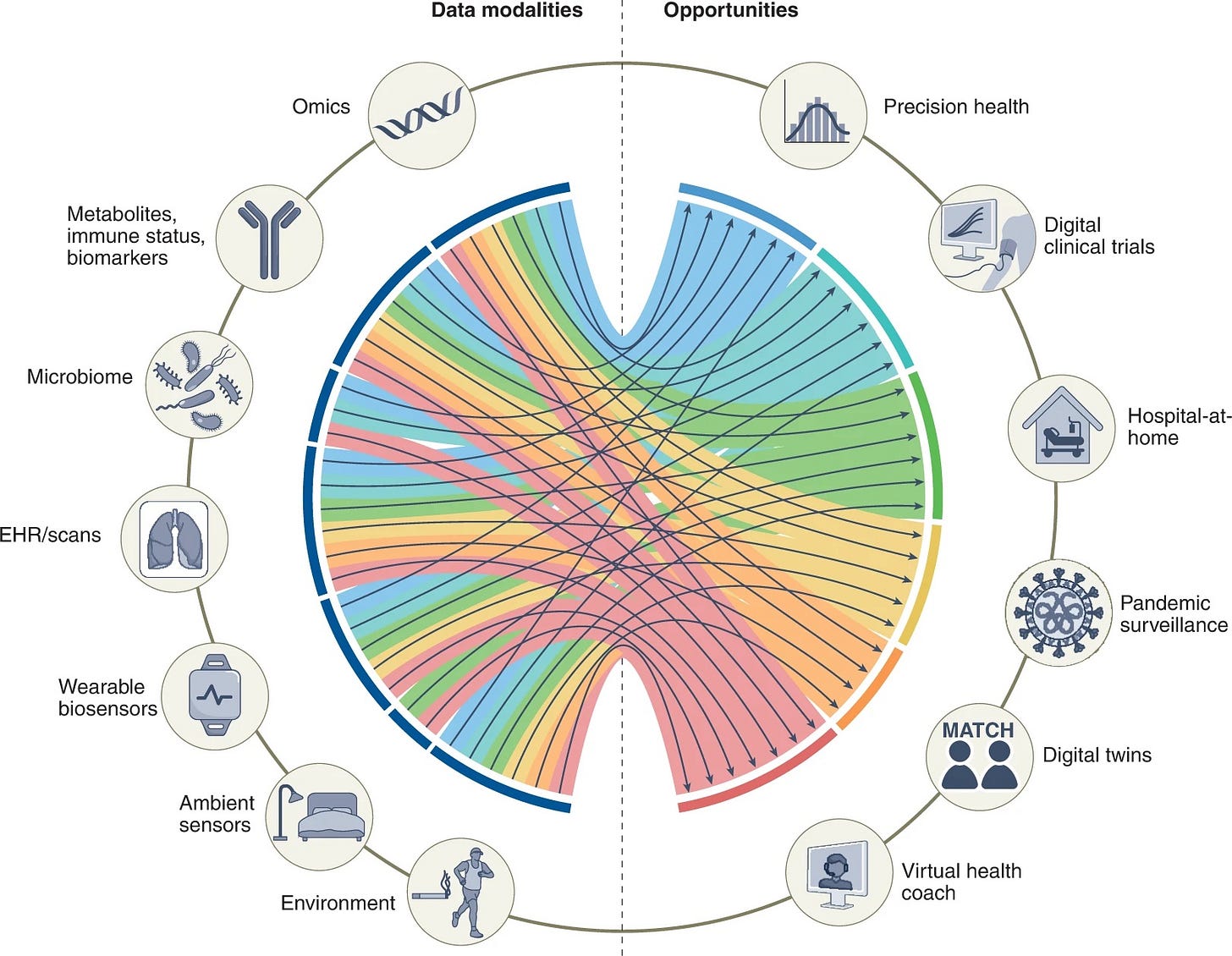
While real-world deployment of multimodal AI in healthcare remains nascent, there are several companies pioneering compelling applications. We’ve seen remarkable progress in vision-language models, evidenced by companies like Theator, which provides multimodal AI for surgery video analysis and annotation of key insights, and RadAI, which delivers AI diagnostics and documentation solutions for radiologists. There is also a groundswell of research in multi-modal AI that has yet to be commercialized, such as chat-based pathology copilots, multi-modal clinical simulators, and self-driving biomedical discovery tools.
When rich biomedical data is combined with population-level information, operational insights, and financial metrics, AI can not only diagnose diseases earlier and with greater precision, but it can also identify factors preventing patients from getting better as well as opportunities for enhancing the function and efficiency of the healthcare system.
Recall that over the last decade, companies like Flatiron Health and Concert AI built billion dollar businesses by combining clinical and claims data.
The new era of multi-modal AI offers a significantly larger set of possible combinations, from pairing multi-omics with clinical, imaging, operations, access, social determinants of health, wearable device data, environmental data, behavioral data, and patient-reported outcomes and more.
Given the new types of data being captured by healthcare AI, the developments in multimodal AI, the resulting ability to combine multiple data sets, and the expansion of modalities available for delivering these technologies, we believe that the healthcare industry stands to benefit significantly from multimodal AI solutions across operational, clinical, and scientific workflows.
3. Vertical-specific infrastructure is needed — and soon
Cybersecurity and data privacy
While representing a thriving market, cybersecurity and data privacy solutions were not built with the unique considerations of healthcare AI in mind. Healthcare data is some of the most sought after on the dark web, and healthcare organizations support a large and ever-expanding attack surface that makes them vulnerable to ransomware attacks, which are notoriously on the rise. Even though healthcare badly needs best-in-class cybersecurity solutions, it’s already fallen behind other industries when it comes to the adoption and strength of its infrastructure due to resource constraints, outdated technology, and tremendous complexity.
It’s possible that the implementation of AI solutions will create new risks, too. AI can expand existing attack surfaces and introduce new types of attacks, such as injection attacks, where the data that a model was trained on is identified. While we’re seeing some startups working on filling these gaps, the infrastructure remains nascent.
Data generation and management
Training healthcare AI models requires high volumes of high quality data that represent intended use cases and population characteristics. Data that comes off as “exhaust” from existing activities within the healthcare system will likely be insufficient on its own (more on the strength and weakness of data types here). Some promising healthcare data marketplaces have come onto the scene in recent years — including Protege, Gradient, and Omny Health — but these alone won’t be able to feed every AI model. The volume of high-quality data is not the only gap, either. Scalable methods of de-identifying sensitive patient data and re-identifying data at scale with patient consent are also needed.
Benchmarking
Benchmarks that measure AI model performance and efficacy are essential for translating research into healthcare products more safely, to the benefit of model developers, AI startups, and healthcare stakeholders of all stripes. Existing approaches have fallen short — for example, attempting to assess ChatGPT’s competence in healthcare use cases by having it take the US Medical Licensing Exam, While thought-provoking, the exam is not a good proxy of model performance on the actual tasks and workflows that exist in healthcare. A legitimate benchmark must simulate the real-world environments that models are deployed in, and their meaning has to be understood by buyers, builders, and users of healthcare AI, as well as the research community.
Model monitoring
AI models can change over a relatively short period of time due to changes in data or the environment in which a model operates. The process, called model drift, can result in the degradation of the model’s performance on certain tasks. Changes in model behavior pose significant concern, especially when AI is addressing critical tasks, such as clinical diagnostics or supply chain management.
For instance, if an AI diagnostic is faulty, the scale of the impact could be thousands of patients (or more) whereas when a single doctor reads a scan, the scale of the mistake is 1:1. Model drift in supply chain management systems could be similarly disastrous, setting off a chain reaction of cascading failures across healthcare networks, and potentially causing critical shortages, resource misallocations, and compromised patient care on a massive scale.
Monitoring solutions have the potential to significantly de-risk these areas by assessing how healthcare models perform over time, and alerting users when there are inconsistencies. Benchmark development is also crucial for effective model monitoring, as well as local validation — a concept emerging through initiatives like AI assurance labs and implementation science centers.
Governance
Safe and effective use of healthcare AI requires a clear owner within an organization. This individual should be aware of all AI solutions being used, oversee their implementation and maintenance, and manage downstream implications. Without such a custodian, responsibility often falls to an amorphous group that may be slow to approve AI solutions, empowered to reject but not approve new technologies, or ill-equipped to oversee proper implementation.
Previous advancements in healthcare, such as the genomics revolution, have demonstrated the importance of having a clear owner responsible for the downstream implications of all emerging technologies, not just AI. In genomic medicine, laboratories conduct genetic tests to identify disease-associated or drug-response variants. But our understanding of variants changes over time. A variant initially classified as benign might later be reclassified as pathogenic, or vice versa, and each stakeholder has to take the responsibility for alerting the next stakeholder in the communication chain: laboratories must update doctors about changes in variant interpretation, and doctors, in turn, must inform their patients. If communication breaks down at any point, it can lead to legal consequences.
The lessons learned from genomics are directly applicable to the implementation of AI in healthcare settings. Recognizing this parallel, some healthcare organizations are proactively creating dedicated roles such as Chief AI Officers (CAIOs) — individuals equipped with a unique blend of expertise in healthcare, technology, and AI. By establishing clear ownership and protocols for responding to AI systems, healthcare organizations can ensure that AI technologies are implemented safely, effectively, and responsibly.
Investment criteria for healthcare AI
Core technology themes
Our initial investment criteria covers six core technology themes for administrative and clinical use cases that encompass what we see as the most exciting areas of work being done today:
Interactive systems: AI-powered platforms or services that enable real-time, dynamic interactions between users and AI algorithms for various healthcare tasks, from clinical decision support to patient engagement and resource management
Multimodal technologies: AI systems that integrate and analyze diverse data types (such as text, images, audio, and sensor data) to provide insights and identify novel patterns
Simulation: AI-powered virtual environments and predictive models that replicate complex medical and operational scenarios to allow for risk-free training, strategy testing, and outcome forecasting
Evaluation infrastructure: Standardized frameworks, tools, and methodologies designed to assess the performance, safety, and ethical implications of AI algorithms across various healthcare applications to ensure reliability and efficacy
Sensor or machine eyes: Advanced imaging, sensing, and data capture technologies that can detect, analyze, and interpret minute details in medical data, operational data, or equipment performance, often surpassing human capabilities
Specialty foundation models: AI systems designed for healthcare-specific applications that leverage large, pre-trained models to augment or automate specialized tasks in both clinical and administrative settings, improving accuracy, efficiency, and scalability across diagnostics, personalized treatment, workflow optimization, and other domains
$1 billion+ healthcare verticals
Healthcare markets may be smaller than they appear, but there are many sub-verticals that are ripe with promising use cases for AI and curious stakeholders, and have TAMs sizable enough to support venture-backed businesses with a $1+ billion TAM. We’ve bucketed a small selection of sub-verticals into lower-stakes and higher-stakes opportunities below. While innovation thrives within these verticals, we anticipate that the most innovative companies will transcend these boundaries, fundamentally redesigning the industry.
As expected, the adoption curve of AI solutions in healthcare is moving from “low stakes” to “high stakes,” or back office to front office and bench sub-verticals. Companies addressing the latter category of opportunities will have to clear more hurdles in order to get to market and face greater scrutiny from buyers. Founders should be cognizant of burn rates and capital needs, given the longer time horizon for revenue generation.
Platform potential
Healthcare’s initial digital transformation — the transition from paper to electronic systems — was hampered by fragmented point solutions that led to interoperability challenges, data silos, and workflow inefficiencies. At first glance, today's AI-driven transformation appears at-risk of similar fragmentation, with Pitchbook data showing over 4,000 US-based healthcare AI companies already in operation. However, a closer look reveals the landscape is markedly different from what we’ve seen before.
Most notably, healthcare leaders, now seasoned technology buyers, have learned from past experiences, and are now much more likely to resist the influx of disconnected point solutions and instead seek out integrated approaches that offer seamless interoperability. To meet the market demand, the most influential healthcare AI companies will have platforms positioned at the epicenter of value creation, not merely impacting a single segment but transforming multiple interconnected workflows across the healthcare ecosystem to drive more systemic and durable change.
We predict that the most successful healthcare AI companies will focus on critical junctures where high volumes of valuable data are generated, upstream of essential workflows.
By owning these pivotal points of data creation and workflow initiation, healthcare AI platforms can exert significant influence over a cascade of downstream processes, ideally capturing the attention of multiple stakeholders (i.e, payer-provider, provider-payer, pharma-provider) and delivering on the promise of a multi-faceted ROI.
The benefits of this approach are three-fold:
1. Workflow control: Platforms can streamline and optimize core processes across multiple stakeholders, and integrate AI-driven insights into daily operations to facilitate standardization and the creation of best practices across the healthcare ecosystem. These changes can significantly reduce friction in cross-stakeholder interactions and information exchange, leading to more efficient and effective healthcare delivery.
2. Data advantages: By building data density with one stakeholder, platforms create new opportunities with other stakeholders in the ecosystem. By capturing diverse, complementary datasets that are unique and hard-to-replicate, they are able to both develop novel multimodal solutions to address complex healthcare problems and increase the platform's defensibility.
3. Distribution: Platforms can leverage existing stakeholder relationships to rapidly scale adoption. As more users contribute to and benefit from the platform, powerful network effects emerge. The central position of these platforms also facilitates cross-selling of additional AI-powered solutions within the ecosystem, while simultaneously reducing customer acquisition costs through an integrated, multi-stakeholder approach.
For example, Abridge, an AI-first clinical documentation platform, has positioned itself at the critical juncture of patient-provider interactions. By capturing and structuring conversation data, Abridge not only streamlines clinical workflows but also creates a rich dataset that can inform various downstream processes, from billing to care coordination to clinical trials, thereby setting the stage to provide value to other stakeholders within the healthcare ecosystem.
In addition to patient-provider conversations, other strategic launchpads for AI integration in healthcare include point of prescription, durable medical equipment (DME) ordering, physician education, evidence generation, clinical trial protocol design, and biological specimen capture and banking, to name a few. Each critical juncture has the potential to become a site of AI-driven innovation and value creation, not just for one healthcare AI company but for many.
Our 40+ year investment in healthcare tech
Bessemer has a long history of investing in healthcare and life sciences over many paradigm shifts, from the genomics revolution to value-based care, and now, to artificial intelligence. Since launching our healthcare AI investing practice in 2018, we’ve partnered closely with domain experts, researchers, physicians, Big Tech, and visionaries across the healthcare and life sciences domains.
What we’ve seen is that healthcare AI is a magnet for passionate founders who want the chance to make a real difference for patients and healthcare professionals, and make lasting improvements to the healthcare system. With so much low-hanging fruit in the healthcare AI landscape, we must also be wary of the "faster horse problem"— the tendency to simply automate or augment existing processes rather than fundamentally reimagining them.
We expect that the companies with the most success, longevity, and impact in healthcare will be those that are able to transform it by re-architecting entire categories through intentional and aspirational design (a process that will certainly require patience on behalf of founders and investors alike).
Potential category-defining companies are already making their market, including Abridge in clinical documentation, Subtle Medical in medical imaging, and OpenEvidence in medical information retrieval, and Atropos Health in real-world evidence generation. These pioneers are not just making existing processes more efficient; they're reconstructing workflows from the ground up with an AI-first core to enable new behaviors, processes, and methods in healthcare.
We’ve been inspired by the progress made in AI thus far: tackling health literacy barriers in clinical trials, assisting in diagnosis of unsolved genetic diseases, and even helping patients recover their own voices. The best companies and most impactful use cases are likely yet to be built. With research driving this change, the opportunities are boundless. The concept of a "learning healthcare system" has been a longstanding aspiration in the medical field, a system that continuously improves by learning from every patient interaction, clinical decision, and outcome. With the advent of AI, we're not just realizing this vision; we're expanding it, moving beyond a learning healthcare system to a learning healthcare industry.
At Bessemer, we have dedicated $1 billion to investments in AI companies, and we’re actively seeking out promising new partners and helping leaders bring their visions to life. If you’re a founder, CEO, researcher, physician, scientist, technologist, executive or simply passionate about advancing this rapidly evolving field, we want to connect with you!
Thanks for reading,
Morgan
This is absolutely one of the best write ups on healthcare AI I’ve read to date. Interesting points about vertical AI modality and business models.
While you mention chief health AI officers as an emerging role, this role is still in its “figuring things out” mode. I’ve seen various roles own different AI tech, depending on the metrics the tech impacts.
Chief health AI officers don’t often hold budget, too — though I can see this changing over time.